You might be surprised by the time you’re finished reading this.
Why?
Because you’ll learn that machine learning applications are everywhere, and you’re probably using them every day without even knowing it.
So, let’s get started—
Fifteen uses of machine learning, along with examples, are waiting for you below.
Apply machine learning to business intelligence problems. Get a free consultation from our experts.
Interested in how to use software for business? Take a look at these guides:
- 21 App Ideas for Startups
- Top Entertainment Apps for Android and iOS
- App Monetization: How to Make Money With Apps
Machine Learning Applications
Healthcare, manufacturing, agriculture, marketing… We chose the top 15 machine learning uses from these and other industries to show the impact of this technology.
Top Machine Learning Applications
- Dynamic Pricing
- Traffic Prediction
- Speech Recognition
- Smart Manufacturing
- Autonomous Vehicles
- Customer Segmentation
- Healthcare Advancement
- Customer Churn Modeling
- Real Estate Price Prediction
- Content Recommendations
- Customer Support Chatbots
- Product Recommendations
- Plant & Crop Field Monitoring
- Intelligent Video Surveillance
- Social Media Content Moderation
1. Dynamic Pricing
Customers are looking for the best prices. That’s why 55% of them go to Amazon to compare prices while the rest goes to multiple sites. So, setting the right price given market conditions has become a major goal.
But how can businesses adjust prices in real time?
Enter dynamic pricing—another example of machine learning application in business used by about 70% of online stores in the U.S. Dynamic pricing means setting ecommerce prices based on current marketing conditions, in real time.
The technology helps answer these questions:
- What’s the best price for products if we want to host a sale in five days?
- What price should we set if our competitors are having a sale for the same product?
- What’s the fair price for a product, given the current competition, state of the market, and availability?
Similar to other examples of machine learning applications, dynamic pricing is driven by algorithms that process big data. They make price predictions through the analysis of many factors, including customer data, transactions, product reviews, competitors’ prices, etc.
Let’s see an example.
Airbnb offers different prices for different days, showing us how the company predicts demand and availability.
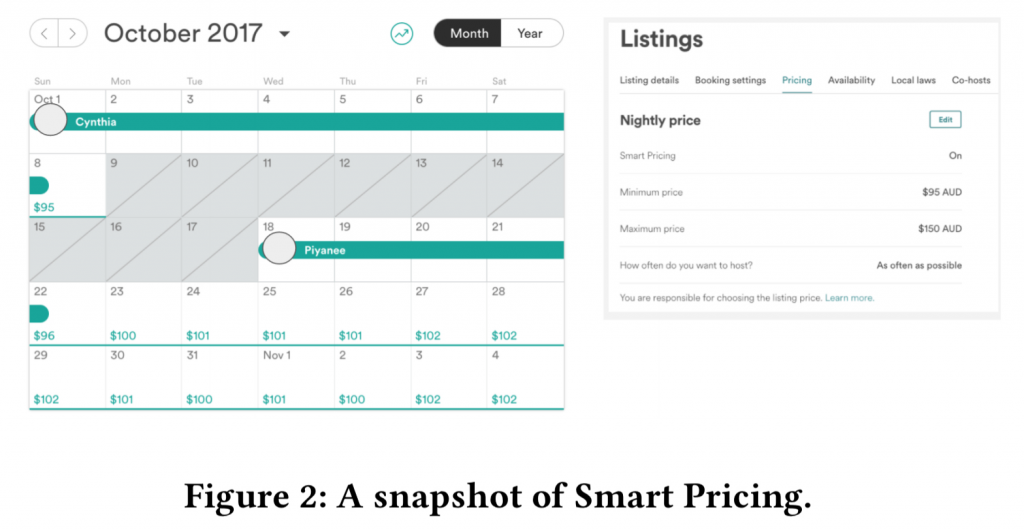
2. Traffic Prediction
Ever wondered how Google Maps knows the fastest routes? Or how does the app locate traffic jams in real time so you could avoid them?
That’s machine learning in action.
Last year, Google finally revealed how machine learning is applied in Maps. The answer: machine learning algorithms that combine live traffic conditions and historical traffic patterns to generate recommendations.
For example:
A pattern can show that Interstate 90 in Massachusetts typically has vehicles traveling at 60 mph between 9 am and 5 pm. But the speed goes down to 30-25 mph around 6 pm. This data is compared with live traffic conditions to make recommendations.
To make recommendations, the algorithm considers many factors, including accidents, road closures, speed limits, road quality, and Supersegments (parts of road networks). For example, an unpaved road covered is not likely to be offered to drivers if there’s a better alternative.
This strategy allows the algorithm to predict:
- Shortest ETAs with a 97% accuracy
- Slowdowns that haven’t even started yet
- Road conditions such as incidents, disabled vehicles on the road, etc.
Here’s the view of the analysis process, as illustrated by Google’s partner on the project, DeepMind.
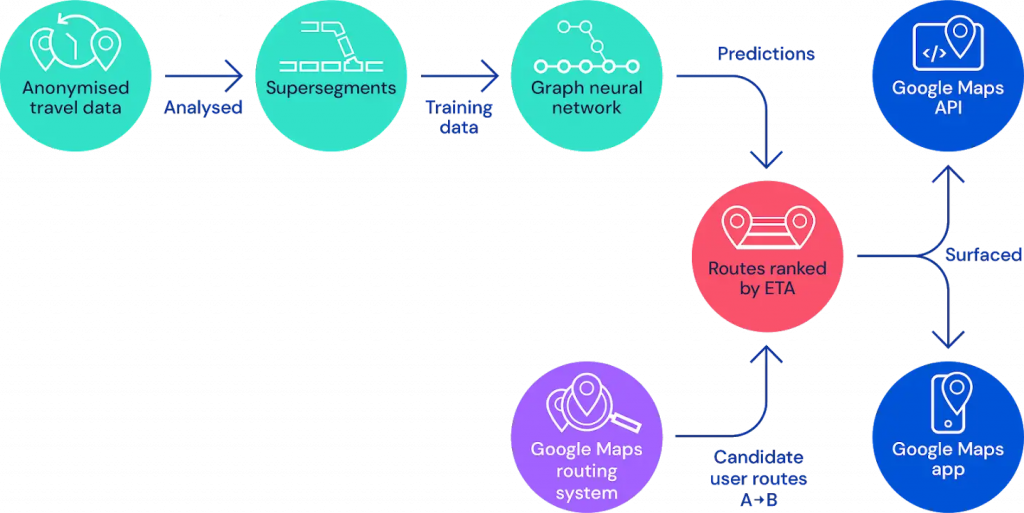
Feel like you want to learn more about machine learning…
These guides should be helpful:
- What is Machine Learning?
- Four Types of Machine Learning
- Machine Learning vs Artificial Intelligence vs Deep Learning
3. Speech Recognition
“Siri, show me a pizza recipe.”
If you used voice assistants or searched for something by voice, you’ve seen this use of machine learning in action.
Speech recognition, the process of converting voice into text, uses a machine learning model to detect speech patterns. The model segments signals into distinct words, helping machines understand what humans are saying.
Technically speaking, the recognition process has four essential steps:
- Audio analysis
- Breaking the audio into parts
- Conversion of audio into a computer-readable format
- Machine learning analysis of the audio to detect words
Speech recognition achieved impressive accuracy, so this application of machine learning is super popular. In the U.S. alone, people use over 83 million Alexas. Combine that with a billion Google Assistant and Siri users, and we’ve got an impressive market.
But this machine learning use is about more than personal assistants.
Speech recognition also helps to:
- Improve pronunciation while learning languages
- Make medical notes in real time to add to patient records
- Enable disabled people to understand content by translating words into text
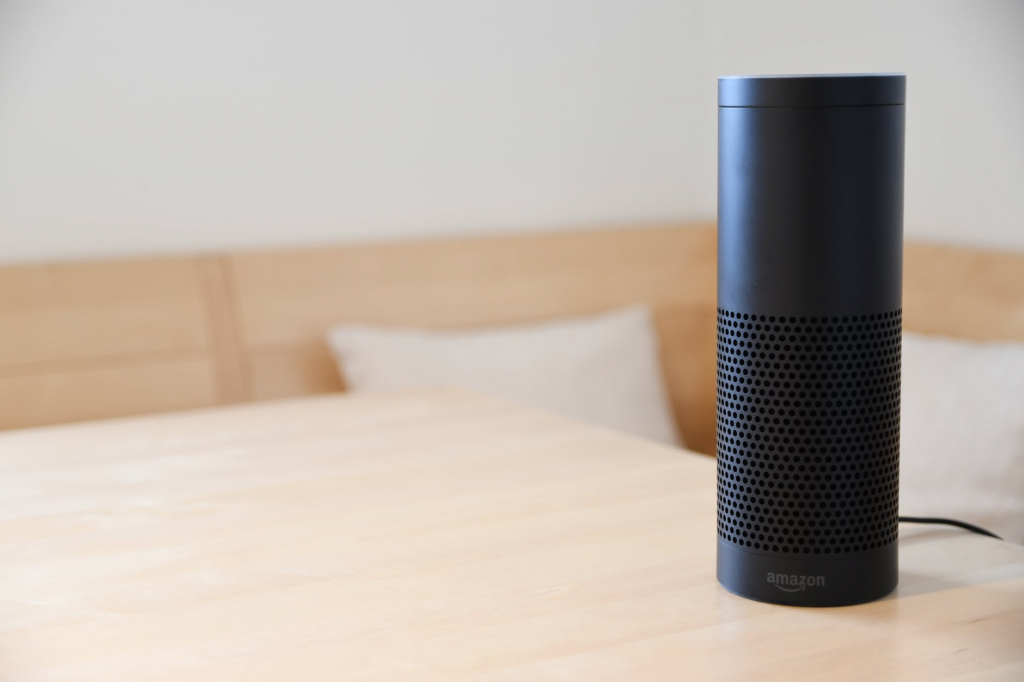
4. Smart Manufacturing
Machine learning is applied in many industrial fields, especially manufacturing. In fact, the technology is behind the so-called “Industry 4.0,” or the usage of IT in factories. Now, for the first time, companies optimize production lines by analyzing performance data from IoT sensors.
Common uses of machine learning in this industry include:
- Industrial IoT. From environment sensors to robots, IoT devices can perform repetitive processes like process monitoring and product assembly.
- Predictive maintenance. Sensors placed on production machines can indicate dangerous wear and errors by monitoring their status and performance.
- Production quality monitoring. Automation of quality control processes with machine learning helps to detect and react to issues and prevent downtime.
These applications of machine learning translate into many tangible business benefits, including increased production output and lower maintenance and labor costs.
Example—
General Electric is applying machine learning to achieve the “digital twin” concept.
A “digital twin” is a digital, data-based replica of a real industrial machine (engine, gas turbine, metal working machine, etc.).
When IoT sensors collect data on the machine’s performance (vibration levels, temperature, noise, output, etc.), they send it to the cloud. There, the data is organized into a model “twin” that replicates the monitored machine’s performance (see image).
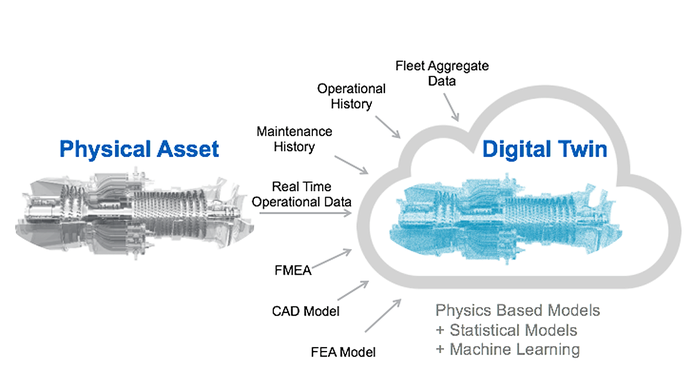
In this machine learning application scenario, the technology is responsible for performance analysis, fault diagnostics, and maintenance prediction.
How effective is the use of machine learning here?
Well—
The digital twin project has been a huge success for General Electric. The data below shows the tangible benefits of the software for the company’s clients. Impressive, agree?
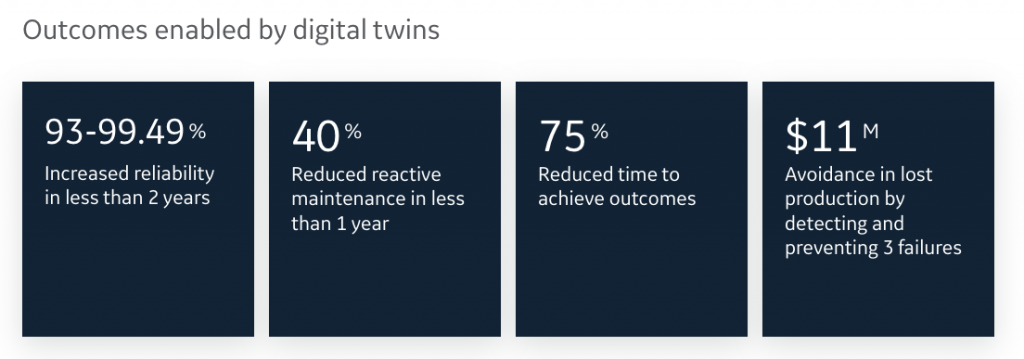
More info on applications of machine learning by production businesses: 5 Uses of IoT Apps in Manufacturing.
5. Autonomous Vehicles
Creating an autonomous car is like building a synthetic animal from the ground up, according to Andrej Karpathy, Tesla’s AI director.
How’s that for an introduction?
But in all seriousness, machine learning applications make self-driving vehicles possible. And the world might need this technology urgently, as 94% of crashes in the U.S. happen because of a driver-related reason.
Self-driving cars can improve safety on roads by eliminating human error.
Many automakers are already working to create a truly autonomous vehicle. The most advanced algorithm comes from Tesla (but is still being perfected). Over 500,000 Teslas feed driving footage back to the company, helping to train the algorithm to detect different objects in a variety of situations.
But how exactly does this technology work?
Autonomous cars use a combination of software, GPS, cameras, laser beams, and radar to navigate their way on the road. Each element is responsible for a certain aspect of driving. This image from Business Insider has every major part of the system illustrated along with their roles.
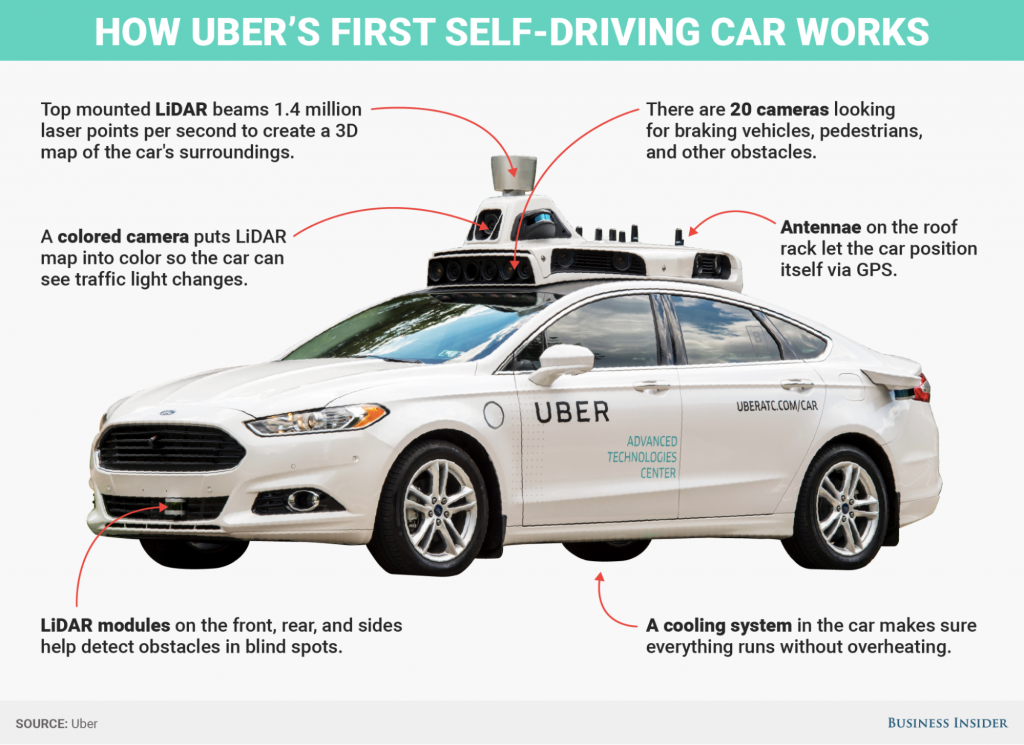
6. Customer Segmentation
Besides setting prices, machine learning applications also help segment customers according to their similarities to achieve more effective marketing. Businesses use prediction models to understand what kind of offers specific customers would appreciate.
Technically speaking, these models look for statistical regularities, trends, and common characteristics in customer data. A result is a number of customer segments with shared attributes, behavior, and interests.
Many companies apply machine learning for this purpose.
For example—
Starbucks analyzes customers’ data and transaction patterns to create personalized offers with its own Deep Brew AI engine. Then, the company deployed those offers to customer segments, including through the loyalty program.
The loyalty program in particular has achieved tremendous success: 23 million members and nearly 50% of the revenues. Kevin Johnson, the company’s CEO, even mentioned that this application of machine learning is a big part of the chain’s success.
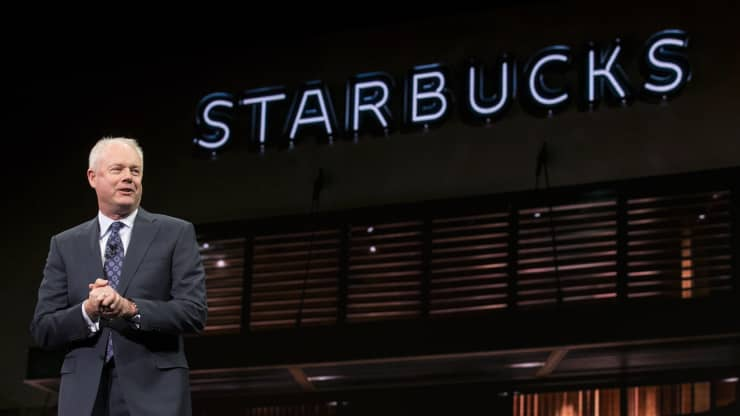
7. Healthcare Advancement
Data is essential in healthcare. Patient records, disease research, drug efficacy testing—the number of potential applications of machine learning is significant. And, major progress has been made to use the technology successfully.
That’s why we’ll describe multiple uses of machine learning in healthcare industry:
- Personalized service. Medical platforms use machine learning to match patients with healthcare providers based on their symptoms and other info.
- Disease identification. Machine learning algorithms can analyze patient conditions and outcomes and generate potential diagnoses.
- Clinical decision support. This machine learning application has been proven to optimize the choice of medications, the timing of treatments, and drug dosing.
- Prediction of patient outcomes. An algorithm containing variables such as diseases, age, characteristics, lifestyle, etc. can predict health outcomes. In this study, a machine learning algorithm was 92% accurate in predicting the mortality of COVID-19 patients
- Improvement of personalized medicine. Machine learning models are 80% better than traditional treatment response prediction methods.
Now—
Let’s see a case study of this machine learning application.
El Camino Hospital used machine learning to analyze electronic health records, nurse call and bed alarm data to determine patients at high risk of falls.
The result was an alert app that notified nurses when a patient was at a high risk of falling. The app helped to reduce falls by 39%.
Cheryl Reinking, CNO at El Camino, has more details.
Curious to know more applications of machine learning in healthcare? Check out this guide: How Machine Learning Transforms Healthcare
8. Customer Churn Modeling
Another business application of machine learning. This time, businesses use this technology to predict customers who are likely to churn, and apply techniques to keep them.
How does this work?
Simple:
You add customer data into a machine learning model to get predictions of churning. The data can include demographics, spending history, satisfaction rating, and other metrics businesses have in their CRMs.
If trained, the model can also create a profile for an individual customer based on what you know about them. You can use the profiles to create personalized offers to try and retain customers.
But the benefits don’t end there.
The full list of benefits from this application of machine learning includes:
- Finding at-risk customers. Machine learning determines customers who are likely to churn based on data about those who already left the company.
- Identifying customer pain points. The analysis of customer behavior can help find specific patterns and problems that lead to churn.
- Helping to define effective retention tactics. Knowing pain points is a good start to make personalized offers to prevent customers from leaving.
Since customer churn is an ever-present issue, companies in many industries apply machine learning to retain customers.
Banks are a good example.
With the customer churn rate in the industry being as high as 25%, banks like UBS, the largest Swiss bank, apply machine learning to detect at-risk clients.
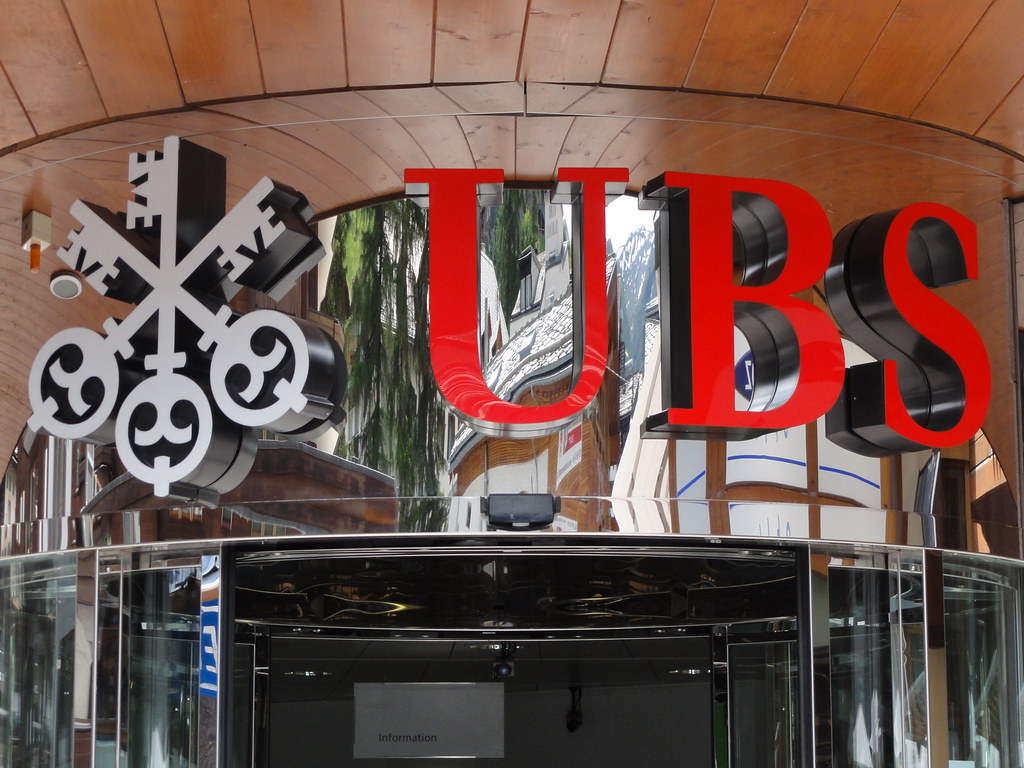
9. Real Estate Price Prediction
One of the most interesting machine learning business applications, proven to be useful. Real estate is the world’s largest and most complex asset market, so any insights are welcome.
Application of machine learning here can help with these questions:
- Which real estate should I buy to maximize returns?
- Where should I buy real estate and when?
- When can I expect the price to be the most reasonable?
As with other uses of machine learning, we get insights by analyzing historical data along with a range of metrics that influence prices. The metrics are basically factors related to properties and their location.
Examples of metrics include:
- Zip code
- Floor area
- Population density
- Duration of construction
- Currency exchange rate
- Estimated construction cost
- Property prices of nearby developments
- Distances to restaurants, food stores, etc.
- Average rating of cafes, restaurants, etc. within a mile
- Proximity to schools, shopping centers, recreational areas
Added with data on thousands (or even millions) or real estate transactions in target areas, these metrics allow machine learning to make good predictions.
How good, you ask?
The difference between a machine learning-generated price and a realtor price might be as low as hundreds of dollars.
And, the accuracy of predictions stays high even if two similar buildings from the same area are involved—the algorithm just needs more non-traditional metrics. Take a look.
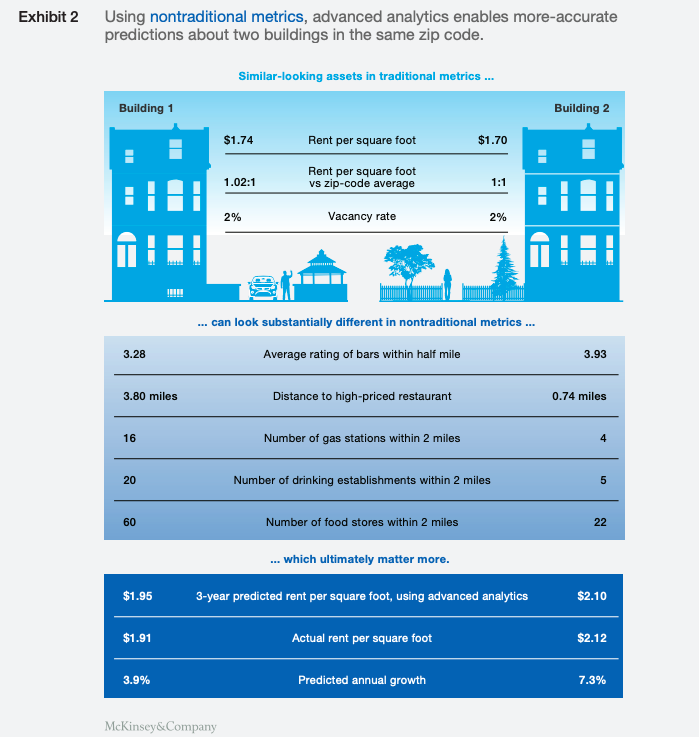
10. Content Recommendations
Have you ever thought about why Netflix content artwork changes when you log in to your account? One day, it’s the entire crew of Money Heist while the other day it’s The Last Dance with Michael Jordan making his iconic Finals shot.
Once again, there’s machine learning at play here. Similar to products, content recommendations are based on the analysis of:
- User demographic data
- Content users searched for, browsed, watched, and rated
- Date and time of watching content
A machine learning algorithm combines these and many other points to create personalized content recommendations. And the more the system learns about the user, the more relevant suggestions it gives.
Since Netflix is a leader in this application of machine learning (that helps the company to maintain 74% of users), here’s a video with their data engineers explaining recommendation algorithms.
Ever wondered what it takes to create an app like Netflix?
We describe the process here: How to Develop an App like Netflix?
11. Customer Support Chatbots
Customers demand round-the-clock service.
Because of this demand, chatbots are becoming popular among businesses. Chatbots are programs that simulate human conversations, typically via a text-based messaging format. As support “agents,” they automate and speed up routine processes.
AI-based chatbots use natural language processing (NLP) and machine learning to understand the input from users. This process takes seconds, so response time is fast (and one of the reasons why almost 40% of people prefer chatbots to agents).
Machine learning allows chatbots to become better at responding with each conversation with a human. The algorithms determine the best responses and use them to be more accurate and helpful next time.
Many businesses are using chatbots for sales and marketing. NIVEA’s Sun Consultant is one of the best examples, advising customers on products and giving skin care tips.
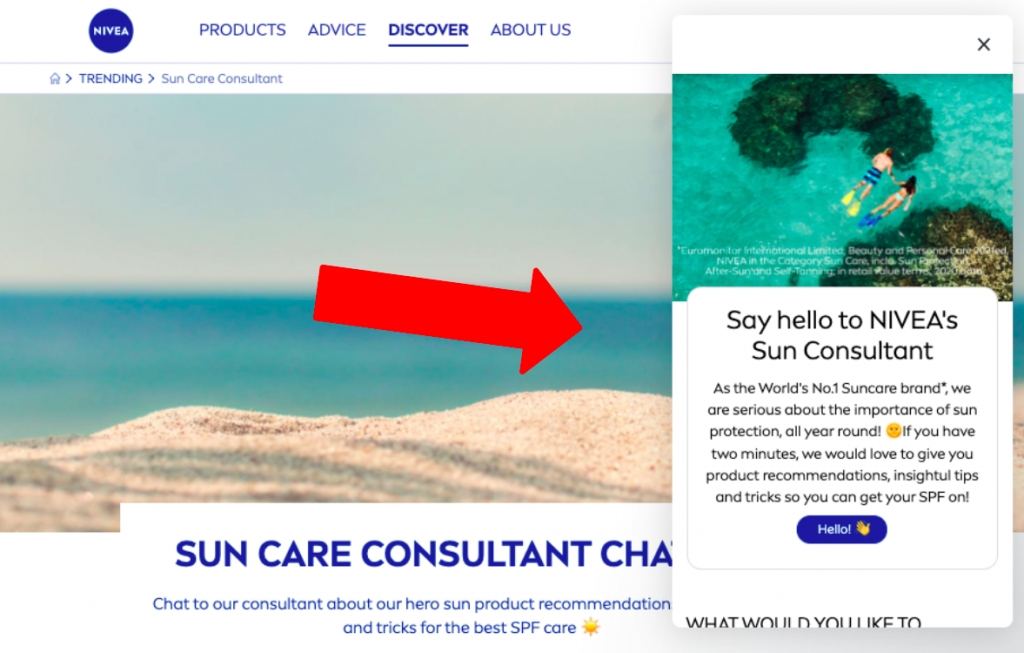
12. Product Recommendations
Large ecommerce retailers including Amazon use real-time machine learning algorithms that recommend products to customers based on their searches. That’s why we see “related” or “similar products” sections when we browse product pages.
Here’s how recommender systems work:
- Step I: User data capture. Algorithms capture information about the users, including browsing behavior, browsing data, demographics, product ratings
- Step II: Contextualization. The information is combined with other customers’ behavior data (how they converted after browsing your site) to clarify the intent
- Step III: Generation of recommendations. Contextually relevant product recommendations are generated based on the patterns found in the customer behavior analysis
A recommender system completes the three steps in a matter of seconds, so businesses can provide each visitor with more relevant browsing experiences.
Amazon is the best example of this application of machine learning in business. There were claims that 35% of the company’s profits were obtained thanks to the recommendation engine, which translates into millions of dollars.
Other businesses are also invited to try Amazon’s recommendation engine. The retail giant offers them a machine learning model called Amazon Personalize that generates recommendations based on customer data.
Here’s a simple overview of how Amazon Personalize works.
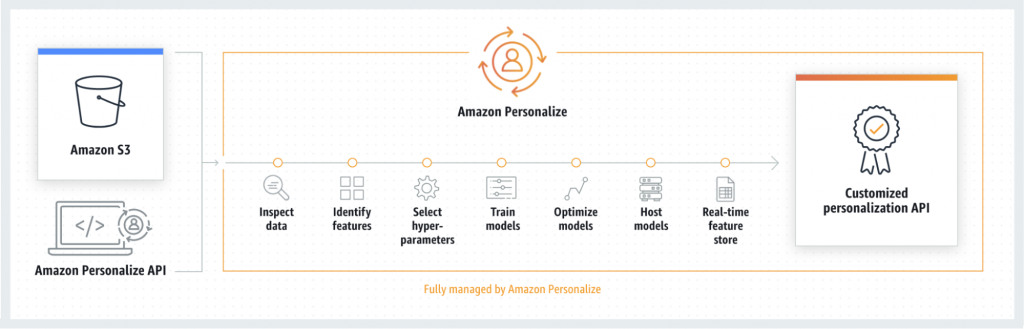
13. Plant & Crop Field Monitoring
AI and machine learning are nothing new for farmers, either. Applications of machine learning in agriculture help increase profitability by increasing yields, reducing the need for manual labor, and raising production.
Monitoring of fields is an interesting case because it helps tackle the goal of increasing crop yield by 70% to feed the growing global population.
Here, machine learning is used to:
- Access irrigation needs
- Detect pest damage in plants and crops
- Save resources like water and fertilizer
Let’s take pest damage as an example to show this application of machine learning.
First, an AI model is trained using images of both healthy and affected plants to recognize the damage. Next, a drone carrying an AI-capable camera is deployed for regular field monitoring. The camera takes pictures to “feed” the model that analyzes them pixel by pixel.
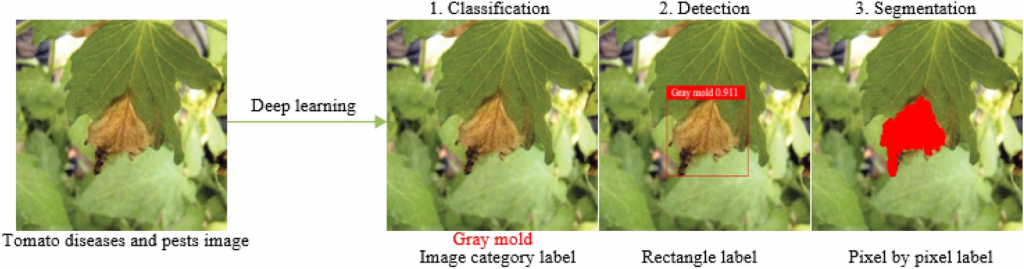
The results of the analysis allow agricultural businesses to identify areas with damaged plants. If farmers take quick action, they can prevent further effects on the yield.
Another application for machine learning: assessment of irrigation needs.
This process includes:
- Taking aerial footage of fields with drones
- Processing the footage with a machine learning model
- Identifying overwatered or underwatered areas
A typical machine learning model employs the leaf water content (LWC) measure to estimate water content and find stressed plants. Many studies have proven the effectiveness of this model, and farmers are using machine learning to improve irrigation.
Here’s an example of aerial footage to be analyzed by machine learning.
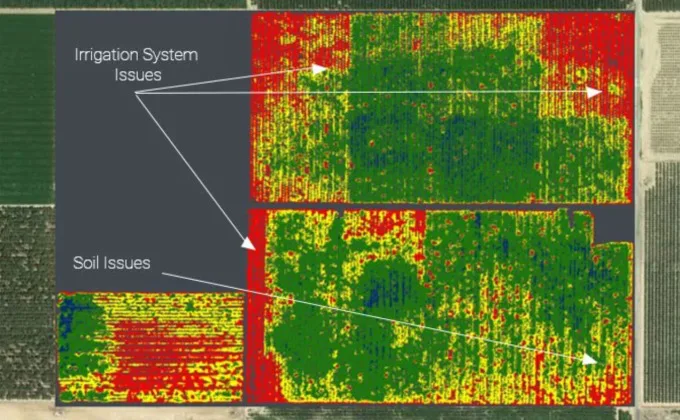
Automatic weeding, plant disease detection, soil health monitoring…. Learn more Use Cases of Machine Learning and AI in Agriculture.
14. Intelligent Video Surveillance
Video surveillance has already become a common application of machine learning. The technology is used to find objects, classify them, and determine their properties. Machine learning has changed the way surveillance works, and added extra ways to increase security.
For example—
AI-supported video surveillance is capable of extremely accurate object recognition and action detection, which is something where traditional surveillance tools are lacking.
That’s why governments and businesses use machine learning to:
- Detect people entering restricted areas
- Identify unusual behavior and dangerous activity in public areas
- Identify possible shooters and other criminals and send real-immediate warnings to the emergency responders
AI and machine learning algorithms can do these tasks without human supervision. Besides, an algorithm can even react faster than a human by triggering immediate alarms.
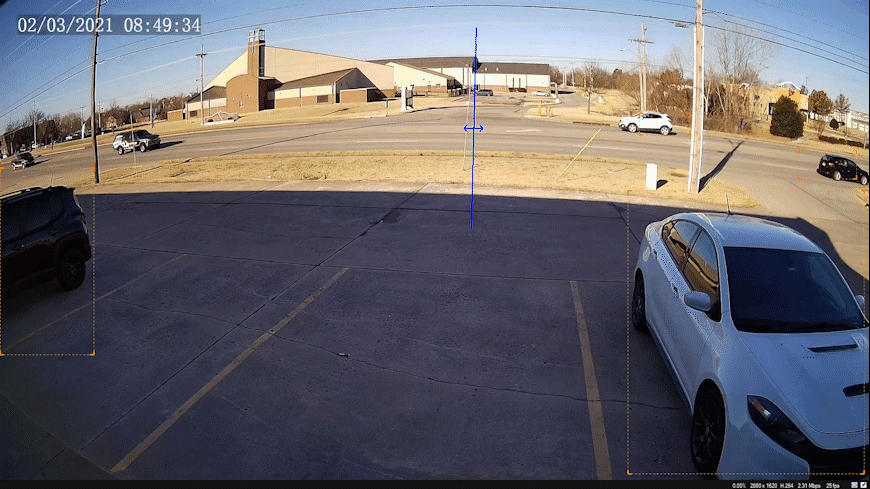
15. Social Media Content Moderation
Social media is the most common place for online harassment. About 75% of Americans said their latest negative experience happened there, and many want social media companies to do a better job at addressing the issue.
The application of machine learning algorithms is one of the ways to remove unwanted content. For humans, it’s simply impossible to go through millions of posts and comments, but it could be a routine exercise for AI.
Here’s how machine learning review systems work:
- Pre-moderation. In this setting, a machine learning algorithm is used before content publishing. If found appropriate, the content will be made visible. In case the algorithm has low confidence in analysis, it’ll request human review.
- Post-moderation. Machine learning is applied after users report harmful content. The algorithm decides whether to remove content and sends it for human review only if unsure about the prediction.
Facebook is a good machine learning application example using post-moderation. Recently, the company revealed the new content moderation workflow, which has machine learning sorting posts for human review.
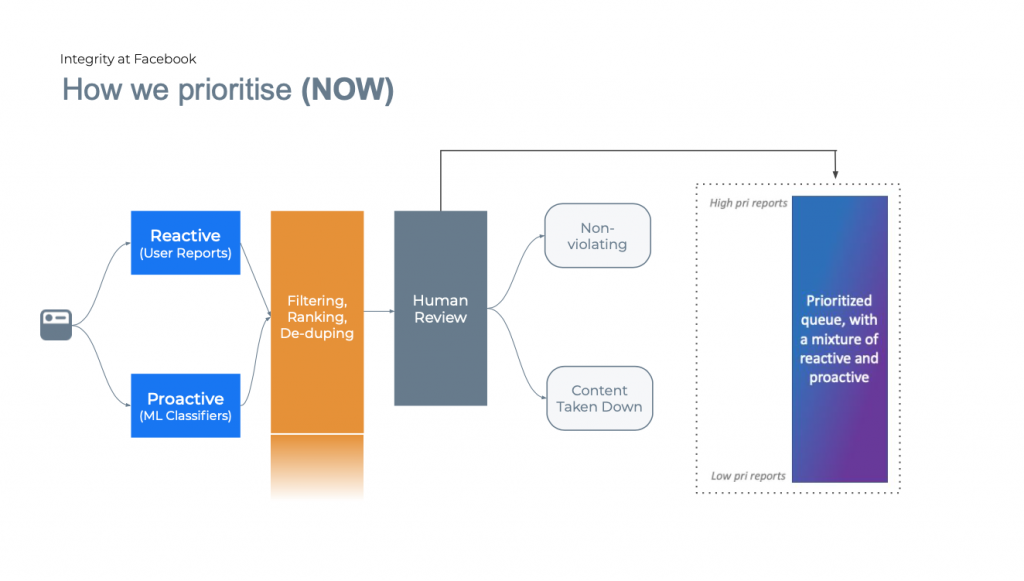
Summary
Machine learning applications are everywhere, from social media content moderation and manufacturing, to ecommerce and medicine. More of us are relying on this incredible technology every day, sometimes without even knowing it.
Once again, the best applications of machine learning:
- Dynamic pricing
- Traffic prediction
- Speech recognition
- Smart manufacturing
- Autonomous vehicles
- Customer segmentation
- Healthcare advancement
- Customer churn modeling
- Real estate price prediction
- Content recommendations
- Customer support chatbots
- Product recommendations
- Intelligent video surveillance
- Plant & crop field monitoring
- Social media content moderation
Get in touch with us if you’re considering building a machine learning solution for your business or organization. Our full-cycle software development company has completed over 200 projects for businesses and has the expertise you need to create top-quality software.