Intro
Did you know that at average, a doctor’s appointment lasts for 17.5 minutes? That’s how long a patient gets to see his doctor, tell him your concerns, and receive the diagnosis and corresponding prescriptions. Taking into account a large volume of information a doctor has to process — the patient’s genome and microbiome, medical history, new symptoms in correlation with these all, and finally medical research to discover a diagnosis — it doesn’t sound like a lot of time. Furthermore, as digitalization continues penetrating various areas of our lives, medical appointments actually get shorter every year since doctors are forced to manually document volumes of information to the computer. Paperwork and hours spent on filling in the software with patients’ data do not add points to quality care. That’s when ML and its applications come in hand.
Today, we’re exploring the benefits of machine learning in healthcare — a topic that remains a mystery for many even in 2019.
What is Machine Learning in Healthcare Context?
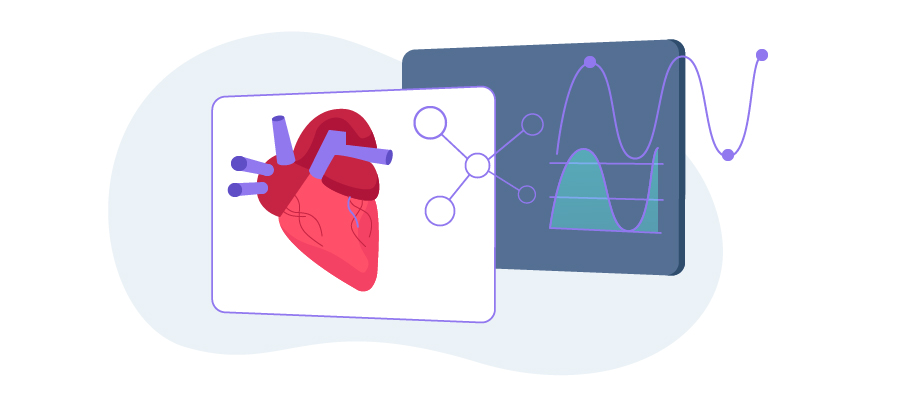
Generally speaking, machine learning is when a machine is able to analyze data and recognize patterns as findings of the analysis. The key difference of ML from traditional computing is the fact that in the case of the first one, there are no specific rules or algorithms of data analysis. In fact, people yet don’t know how exactly a machine finds what it finds. The process behind a computer becoming smart is called training. Software engineers load software with a certain data set and make it understand that data through training it until the output is appropriate. Afterward, the software becomes able to digest the data gathered from real life and interpret it itself.
Even though the term deep learning is often matched against machine learning, these two cannot be used without one another. Deep learning is actually just a subset of ML that lies in the usage of artificial neural networks. These networks consist of neurons — numbers that mimic the performance of human brain cells. The network is built in layers, the input layer in the first one where the data is received. The very last one is called the output layer where the network releases its findings. And in between are the hidden layers, this is where the actual data processing takes place. This subset got its name due to these hidden layers inside of it; till these days we don’t know what exactly happens there and how the system comes up with the end results, it’s performance is just impossible to retract and break down into steps, at least for now.
To illustrate one of many possible examples of machine learning in healthcare, imagine software engineers are trying to build a neural network that would detect if a person has skin cancer (also called carcinoma in the medical environment). To make the network able to tell the difference between healthy and malicious cells, the first thing to do is to create a model based on data set of tissue scans of, let’s say, 2000 patients (the more the better) that were already checked by doctors and diagnosed positively or negatively. After that, the network is ready for you to upload an undiagnosed tissue scan. Basing on the earlier diagnosed pieces, it will detect carcinoma symptoms on a fresh sample. Depending on how ample the training data, capabilities of neural network model can expand from “yes” or “no” answers to a prediction of how certain disease is likely to occur in a certain patient. Data useful for machine learning applications in healthcare include:
- Genome — a set of the genetic material enciphered in a person’s DNA.
- Microbiome — a collective term for the billions of microscopic organisms living inside our bodies.
- Clinical record — a patient’s medical information on what’s
- Personal device data gathered by smartphones, fitness trackers, smartwatches or any other gadgets that run health-tracking apps;
- Social information — this includes things that directly influence a patient’s lifestyle, like income, profession, living habitat, etc.
- Exposome — this is a collective name for environmental factors that one way or another affect human health.
Machine Learning Applications
Depending on what information can neural network access for training, it could then become a powerful healthcare software that helps (if not replaces) human medical practitioners. The most actively used learning applications in healthcare include:
1. Diagnosis prediction
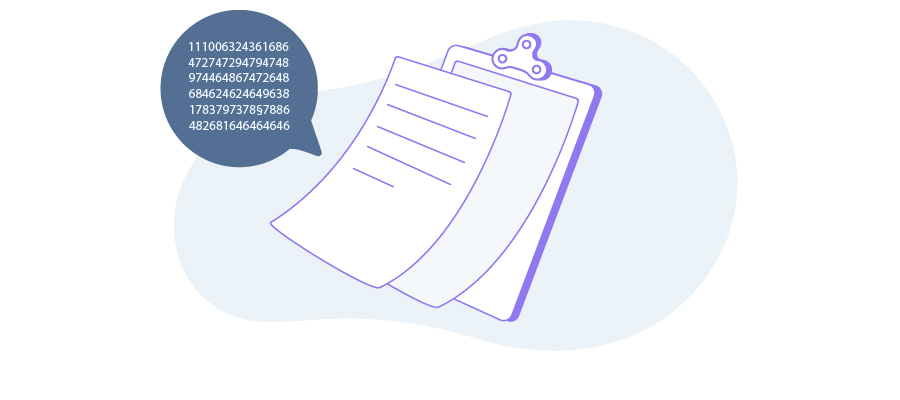
The primary application of machine learning tech stack is aimed at solving the biggest healthcare pain — identifying diseases in patients. You might have already started wondering, why the flu or cold you’ve caught recently was diagnosed as decades ago — by a human doctor within minutes, no high-tech equipment or AI-powered robots involved. Obviously, diagnostic ML software is applied to the hard-to-diagnose states, not some random and common complaints. Hard-to-diagnose obstacles include mostly genetic diseases like cancers that show no symptoms at the very beginning when it’s easier to treat, auto-immune disorders, and other states with atypical symptoms and of unknown origin.
A real-life example of AI solution for diagnostics is the well-known IBM Watson Genomic. It uses cognitive computing and deep learning to detect molecular pathologies in patients. It also improves itself without any supervision simply by extracting recent information from peer-reviewed literature. Altogether, this allows Watson Genomic to provide not only quite accurate diagnosis predictions, but even suggest targeted therapy options forming the precision oncology care program.
2. Diagnosis Visualization
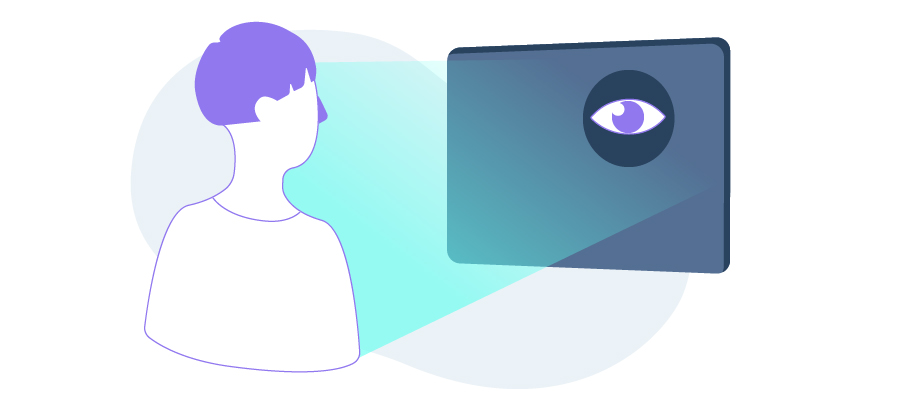
ML-powered diagnostic wouldn’t be as revolutionary as it is without another subset of artificial intelligence called computer vision. In short, this scientific field deals with computer programs analyzing images and videos and understanding what’s depicted there in a way a human eye and brain would do. Computer vision here is used to create three-dimensional radiological images that would provide a fuller picture to the health state of a particular patient. The InnerEye project is aimed to provide doctors with:
- accurate radiomics measurements for quantitative radiology;
- easier radiotherapy planning;
- precise navigation during surgeries.
3. Personalized and precision healthcare
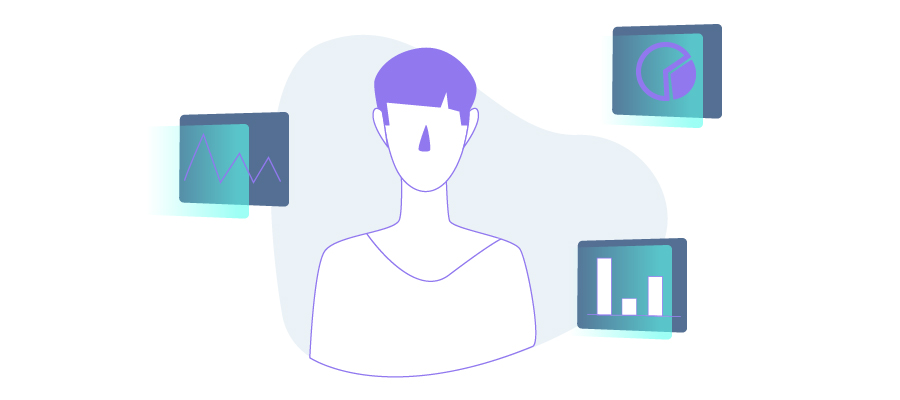
Disease assessment advancements and shorter in time medical research are only a few factors that increase the value of machine learning in healthcare projects. Scientists work hard combining all the numerous subsets of AI, that include natural language processing, robotics, computer vision, deep learning, and cognitive analytics, to make the precision medicine practice possible as soon as possible.
So far, doctors have been working with a limited set of tools, whether it be for diagnostics, therapy, preventive care or health risk estimation. But ML applications listed above already position the industry for major changes, the main being an idea that certain type of cancer in one patient is different from that same type in someone else. Meaning, a doctor doesn’t just diagnose it and then follow a therapy pattern but applies necessary ML subsets to examine cancerous cells in a patient and precisely treat them.
Disease intensity as a factor oftentimes is left ignored by practitioners, which can later result in an ineffective therapy or harsh side effects and general health decrease in a patient. Machine learning-powered apps, in this case, allows to gather and thoroughly analyze a wide range of personal data from genome and exposome factors to a prediction about how the body would react to the therapy on a cellular level.
Gene-editing technology is another just as revolutionary example of precision healthcare. It lies in the ability to explore a patient’s DNA and choose certain genes to activate or inhibit certain genes. Believe it or not, gene-modified people don’t belong to the fictional X-men universe only. Namely, at the beginning of 2019, Chinese genetic scientists created the world’s first genetically modified baby girls, whose genome was changed in a way that made them invulnerable to HIV. Exploration of the genome sequence for that experiment wouldn’t be possible if not for certain ML applications used by Chinese scientists. Although this sounds mind-blowing for many, the experiment was also highly criticized by society due to ethical and moral reasons, and as a result, the interest to it stopped being so vocal and public. Anyway, we couldn’t agree more that AI transforms precision medicine and personalized healthcare in some beautiful ways giving hope to those who had literally no chance to cure just a decade ago.
Summary
It wouldn’t even be an over-exaggeration to say that applications of machine learning to healthcare issues have limitless potential. AI is taking the world by storm penetrating into literally every business out there, and it’s not going to hold back anytime soon. Regardless of what industry you’re working in, we bet your company would benefit from quality custom ML application to solve its business challenges whatever they’d be. Sounds interesting? Click here to start growing with IDAP.