Supply chain modernization & digital support have never been more urgent than now. The industry is experiencing global transformation and requires innovative business solutions. Therefore, companies implement various technologies to speed up supply chain recovery from the pandemic and the war. Another innovation enterprises prefer for this purpose is Machine Learning (ML) – a branch of AI enabling the training of a computing model on observations or data.
Following Gartner’s predictions, by 2026, over half of supply chain organizations will use ML to augment decision-making capability. But it’s just a tiny part of how Machine Learning can improve supply chain efficiency. We collected different use cases to help you consider ML as a real lifeline for your supply chain reality. Scroll down to explore why Machine Learning is worth your attention and investment in 2023.
Related: What is Machine Learning and How Does it Work?
Why the global supply chain needs renovation
Supply chain companies always face challenges regardless of time. It’s a dynamic niche. In plain language, you must ensure proper item delivery from the manufacturer or miner to the end user. Fighting with pitfalls is perennial here. Meanwhile, now the scale of difficulties leads to unexpected and harmful consequences. Companies should find a versatile solution for several urgent issues, such as:
- Logistics disruption
- Unoptimized routes in unstable geopolitical conditions
- High operation costs
- Last-mile delivery issue
- Lack of experts in the supply chain area
Poor customer experience, downtimes, delays & breaching B2B contracts are just a part of the outcomes the above problems can provoke. They can’t be covered with manual effort, assets, and Google Spreadsheets. Therefore, business leaders opt for technologies like ML, AI, and IoT to predict what will happen next and know how to protect companies from new pandemic- or war-like disasters. According to KPMG research, 67% of CEOs admitted they would increase investment in disruption detection and innovation processes.
The conclusion is that the supply chain we’re used to cannot exist anymore. It doesn’t match time and demand. The future belongs to the flexible and digital-oriented supply chain, and Machine Learning helps companies build it.
Related: Technology in Supply Chain Management: How Innovation Boosts The Industry
Top 7 ways how Machine Learning can improve supply chain efficiency
Improving Demand Forecasting
The crucial component of the supply chain is proper planning since it can help avoid possible pitfalls, increase overall business performance and cut costs which is on the agenda now. Traditional demand forecasting in this area requires time-consuming manual work to process vast amounts of data and predict customer demand.
Meanwhile, Machine Learning allows businesses to simplify this process and makes results more accurate. It’s capable of analyzing Big Data regarding customer behavior, dynamic market conditions, etc., to provide you with a clear picture of what and when end-consumers will need.
Ericsson reports that to perform a digital-oriented data analysis, Machine Learning uses several types of information, such as:
- Previous demand
- Confirmed future sales
- Product data
- Customer information
- Pre-sales data
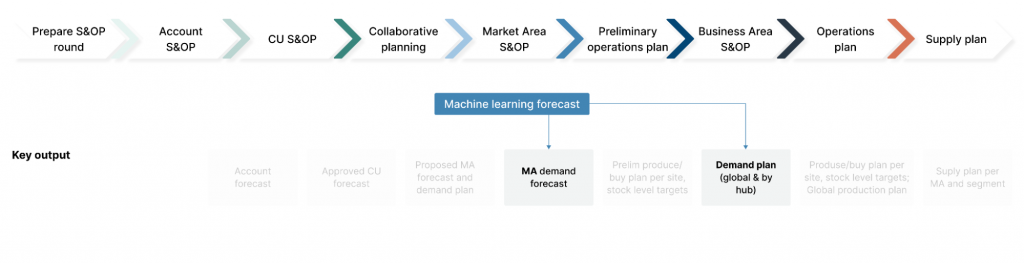
Inventory Management
An efficient supply chain requires organized and smooth warehouse operations as well. ML-based inventory management strategy helps companies track goods’ location and storage conditions, the number of involved employees who handle order preparation, etc. Machine Learning here applies to companies with more than one warehouse in different parts of the world. Business leaders use this technology to synchronize operations and implement new optimization strategies more efficiently.
Modern inventory ML-based management systems also include robotic technologies. Precisely in this area, mobile robots replaced employees. Just consider the experience of Amazon, Walmart, and other retail giants. Now, Amazon has 520,000 robotic drive units which manage packing, in-house delivery & lifting, etc. They show shocking efficiency and productivity. Moreover, robots here reduce the number of human mistakes and eliminate room for fraud which is also essential for businesses.
Optimization of Logistics
The core supply chain weakness today is routes. Companies have been facing this problem for several years, starting from COVID-19 times and ending with the russia-Ukraine war. Governments implement restrictions that shouldn’t be broken. Consequently, legacy routes become more costly and time-consuming. It makes companies think about logistics optimization, and business leaders apply ML to cope with this process without pain & suffering.
Machine Learning changes route maps in real-time, considering various factors that can slow down the delivery process. The technology analyzes input data and compares it with explanatory variables to create accurate data models and work following results. It helps choose the shortest routes and reduce delivery costs.
The more data ML processes, the more accurate optimization it makes. Consequently, since ML has already done it, employees don’t need to make any risky decisions or actions to improve their supply chain.
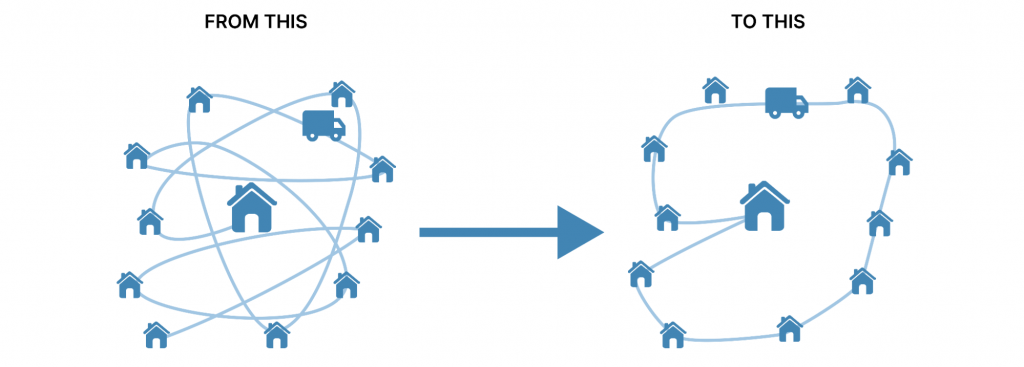
Related: Digital Twin for Supply Chain: The Core Reason for a Post-Pandemic Recovery
Predicting Preventative Maintenance
Another troublemaker for supply chain companies and manufacturers is unexpected hardware failures leading to downtime. Forbes reports that 82% of companies have at least one unplanned downtime per year, costing about $50 billion for manufacturers. Most likely, the exact amounts apply to the above supply chain businesses. What can Machine Learning do for your supply chain here? It analyzes real-time data and sends relevant alerts about possible failures to help fix the issue before it arises. This way, Machine Learning saves vehicles, hardware, and other assets companies use to support the supply chain. For businesses, it means:
- timely delivery
- proper fulfillment of contract obligations
- better end-consumer experience
- reduced supply chain costs
- cut maintenance costs
- elimination of manual work
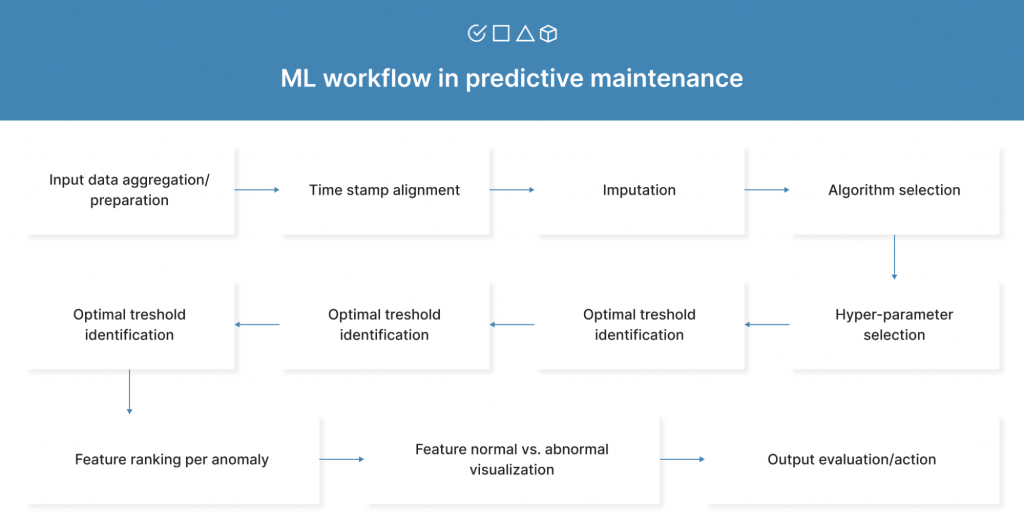
Reducing Fraud Risks
The supply chain experiences fraud too frequently. Usually, companies can’t track their shipments properly or can’t see how employees perform their duties in warehouses, etc. Unfortunately, this area isn’t about trust since it comes to significant investment and process complexity. Therefore, to protect assets, companies implement Machine Learning. Supply chain becomes highly secure since end-to-end transparency allows business leaders to track each step and route. Machine Learning uses risk rules and compares them with the input received. In case of inconsistency, it provides real-time alerts to employees.
Related: TOP 10 Logistics and Supply Chain Applications
Automating Quality Inspections
Quality inspections require Machine Learning in supply chain. Case study that we’re used to considering here is the ability of machines to evaluate the packs to detect some defects if so. This routine work makes people tired, so they can’t provide a 100% guarantee that all items are correctly inspected and packed. Meanwhile, any damage to the packaging means a breach of contract and, most likely breach of storage conditions. By analogy with previous cases, hardware compares real-time data with previously set up standards and concludes the quality of packaging.
End-to-End Visibility
The supply chain looks like a labyrinth with unlimited stakeholders, routes, items, and steps to deliver shipment from point A to point B. Sometimes even this simple path, which seems to us a straight line connecting two points, can pass through other points: C, D, E, etc., to cover a larger area and deliver more in a short period.
Naturally, companies lack supply chain transparency, and it causes lots of issues starting from high delivery costs and ending with breaching strategically essential contracts. Machine Learning allows business leaders to track each stage of the supply chain and provides real-time data round-a-clock to show each detail of the process.
This way, enterprises can be sure their plans and strategies match reality or define core challenges to eliminate them. In other words, regardless of supply chain complexity, ML can turn it into a clear and transparent line for all stakeholders between point A and point B.
Summary
If you have to operate a wide network of suppliers, storage points, transport and stores, supply chain management can become a challenging task. Luckily, technologies such as machine learning can help you at all stages. The advanced algorithms can accurately predict demand, optimize logistics, help you efficiently manage inventory, and automate inspections. All these factors will contribute to an end-to-end visible network that will be less vulnerable to fraud, work with more efficiency, and require fewer operational costs.