Intro
These days, Machine Learning is always discussed in the context of valuable user data insights retrieval. Getting to know your users, learning their tastes and interests is a proven key to customer loyalty, which leads to all kinds of benefits like sales and brand awareness. But is there a place where loads of users’ data are awaiting for businesses to be collected and processed? Sounds too good to be true, but actually there is — social media. Today, we are exploring ways Machine Learning is used in all kinds of social media, both by media owners and third-party companies hungry for customer insights.
Social Media Monitoring
Social media monitoring is a process of exploring and analyzing what has been said about a company, its product or service on the internet, primarily online resources dedicated to socialization purposes. Years ago, when social networking wasn’t as widespread as it is now, brands were able to keep up with users online simply by scrolling the feed and checking tags and comments. But as people developed a habit of posting on their socials daily, if not hourly, the amount of data proliferated at a rapid scale and became impossible to process manually. Luckily, the introduction of effective ML techniques for data processing gave us the automation level we needed.
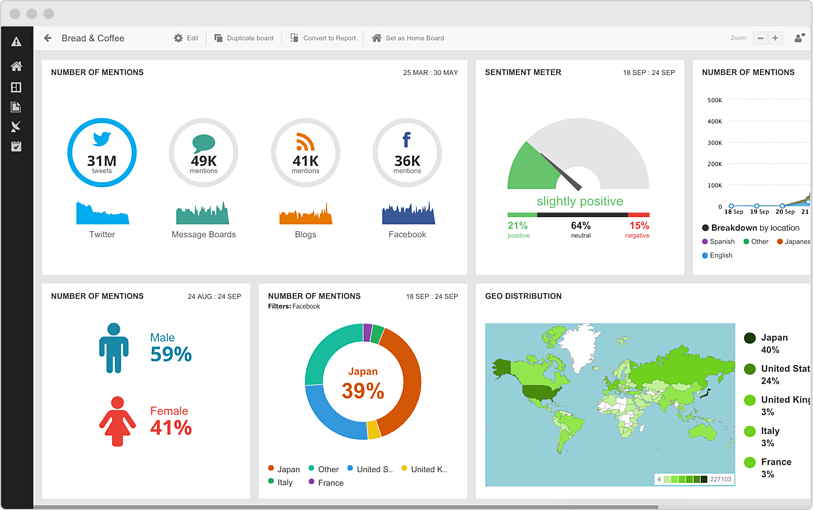
With automated ML-powered solutions for social media monitoring brands finally get access to actionable insights to manage their brand image effectively and find out users’ feedback (even when it was not meant to be heard initially, which is the best part of the whole thing) timely. In short, it works as follows: Machine Learning-powered network analyzes text, images, audio, and video files found on social media detecting mention of a brand being it a logo on a photo, a typed-in name, or a pronounced name in the video. That ML network obviously does not exist on its own, it is usually a part of a ready-made or custom social media monitoring platform. Among some popular digital products of that type are Youscan, Keyhole, Hootsuite, Digimind, and Sprout Social. Some of them are richer in features compared to competitors, but the core functionality is basically the same: brands (as users) can track social media activities, from brand name mentions to keywords perception. Most platforms provide the options of automatic reports, timely reminders of new or unusual activity on the web, and safe data storage as well. The fact that social media monitoring has become surrounded by a corresponding software, proves that brands highly rely on it as a part of their PR and sales strategy.
Social Media Marketing
Social media marketing is the use of social networks to promote a brand, its product or service. Even though it technically remains a part of digital marketing, the exponential growth of social media resources makes researchers and practitioners separate it into a standalone discipline. Last year, Gartner predicted 30% of companies will entrust at least one of their sales processes to AI by 2020. Well, we’ll definitely check that prognosis at the end of the year, but even predicted numbers say it all about the ML benefits for business. The key goals of digital marketers — personalization, analytics, optimization, brand awareness — can be taken to the next level quite easily incorporating Machine Learning solutions into the company’s operations.
First and foremost, Machine Learning allows brands to drastically scale personalization, regardless of the sphere, product or service. Years before ML hit the industry, by calling a marketing campaign personalized, people meant sending a follow-up email to a person who didn’t make it to the checkout when shopping or delivering a selection of items that he/she looked through earlier. That changed when Machine Learning entered the digital marketing chat. Now, websites and apps can choose what content to display depending on the user’s behavior and interests, and even location. Naturally, personalization goes hand in hand with social media as well — primarily because it’s a proven source of customer insights. Such benefits as optimized advertising, automated marketing campaigns, smart content creation, augmented analytics, automated content alteration, and effective PPC campaigns are only possible if a brand achieves a high level of marketing personalization or, in simple words, got to know who its customers truly are as separate individuals with their preferences, tastes, and interests at this very moment. From the technical side, most Machine Learning applications for social media marketing highly rely on such AI subfields as natural language processing and optical character recognition.
Augmented Social Network Experience
While the business use cases of Machine Learning to manage social media activities can be briefly described as the two major directions as we did above, the ways ML transforms user experience are numerous. We highlighted the most popular ones that in a way revolutionized social networking after entering the market.
Text recognition
It might seem that audio and video messages have taken the world completely, however, the largest part of messages delivered on socials still come in textual form. Naturally, sooner or later social media apps had to find their way to read our conversations. As usual, Facebook pioneered this field as many others, this time — creating DeepText network for text recognition. This is a powerful Deep Learning-based program that not just recognizes characters and puts them in words, but studies the context of what’s been said. Later, the raw data mined by DeepText is used for content recommendations, feed forming, safety measures, and behavior analysis.
Image recognition
Computer vision applications have been used in numerous industries from automotive to construction among others, and over time they made it to the social media industry. The most controversial technology of the Facebook AI suite is the famous DeepFace network for face recognition. A nine-layer neural network with over 120 million connection weights that’s been trained with billions of data samples, it tracks digitized human images and makes the search of a person by a single photo very fast and accurate. DeepFace wasn’t warmly welcomed by users nor by some governments. Namely, the European region forced Facebook to disable this functionality for its residents since it violated personal human rights in the opinion of European officials. Other than that, many people believe DeepFace and other image recognition tools made social media a safer place as these are also applied to detect pornography, violence, and other disturbing content, not just to deanonymize the users for no apparent reason.
Content recommendations
Just like brands that hire whole departments of people that are supposed to work on customer loyalty and retention, social networks as well are occupied with making people stay online and enjoy the time they spent on particular sites or apps. Personalized content recommendations are to blame for the addiction-forming nature of social media. Networks invest enormous budgets in Machine Learning technologies that generate media feeds based on what users like, search for, and — most important — might like potentially. A great example is the Instagram Explore tab, which analyzes what posts capture your interest to pack your Explore tab with similar pics. Also, Instagram applies ML not only to show content to people but hide it as well. After you report an image you don’t like (and it should not be anything forbidden by the platform, just a pic that you personally find disturbing), the application will disable such posts from your feed or will at least ask whether you’re sure you want to see the post.
Neural translation
The benefits of machine translation do not require a long description. However, for years we suffered from machine translation that barely made any sense and was good only to giggle at it in our spare time. As a try to make social media more open and united, in 2017 Facebook introduced a new approach to the neural machine translation (the world neural indicates Machine Learning involvement in the whole thing). The new translation architecture they offered, stood for translation in the context, unlike the methods functioning earlier. Today, users enjoy numerous social media posts in their native language and have an option to communicate with whoever online with an impressive level of understanding.
Population density
Based on the real-time user geolocation data, Facebook creates maps that display the most accurate and up-to-date population density. This might seem unimportant until finding out the task of creating such a map in real-time was practically unaccomplishable in the past. Back in 2016, it started with 22 regions. As for today, it is coming close to providing us with world-wide coverage (except for the areas with no or very minimal population). The maps are used for safety and healthcare matters, like rescue activities in case of natural disasters or vaccination planning and implementation.
Summary
Some people find the application of Machine Learning to social media use fascinating and promising, while others loudly dislike it and refer to George Orwell’s classics discussing the whole face and messages recognition thing. We believe with appropriate work ethics and respect for personal data, Machine Learning in social media can benefit both businesses and individuals greatly. Any thoughts on that rather controversial topic? If yes, click here to start a discussion, we’d be happy to find out your opinion.